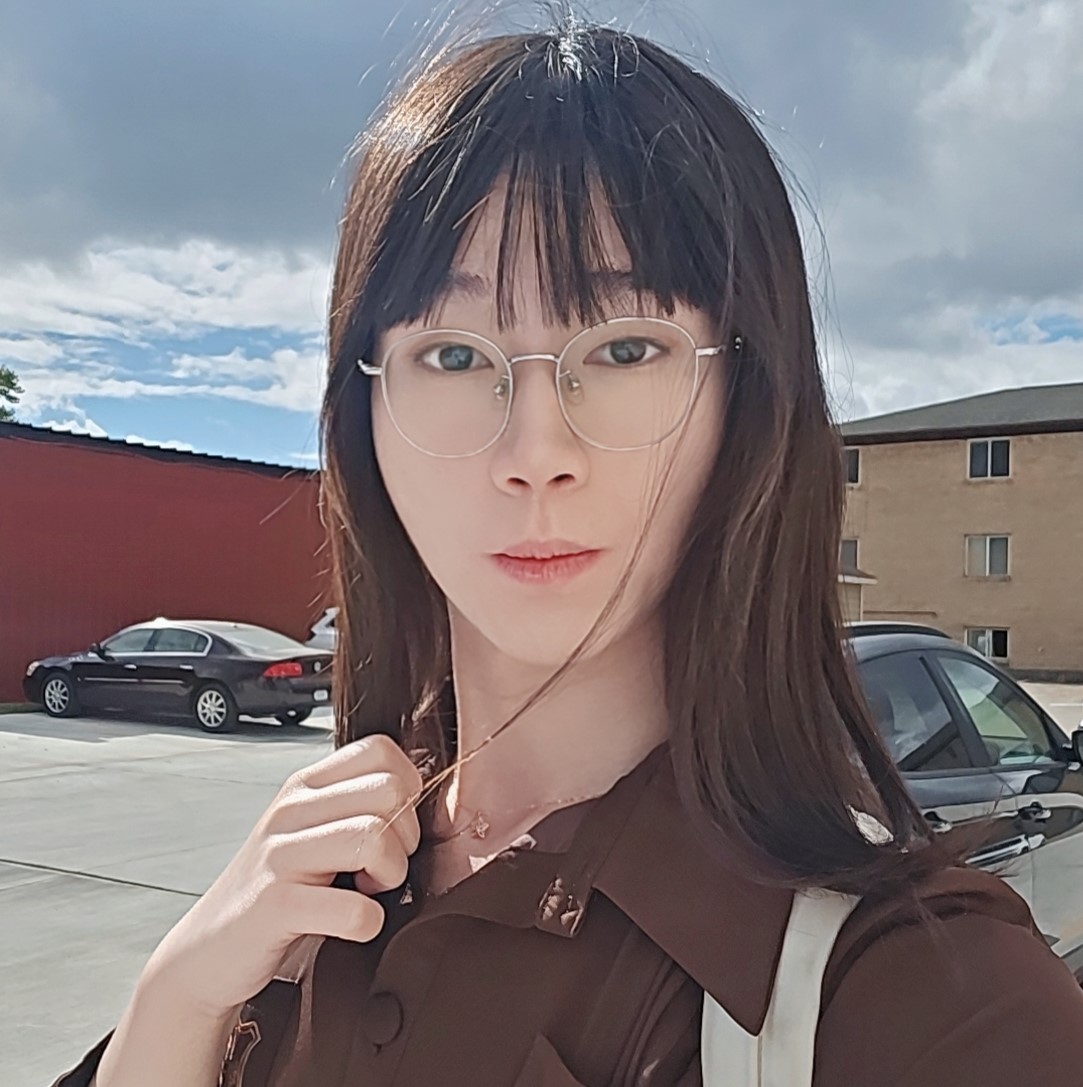
About Me
Hi! 👋 I'm Tianyang Xu, a second-year PhD student at Purdue University (opens new window).
My academic interests lie in the field of Natural Language Processing (NLP) and large language models (LLMs), with a focus on mitigating hallucinations, aligning models with human-centered objectives, and enhancing the overall safety and reliability of these systems. I am interested in exploring innovative approaches that ensure the effective use of LLMs in real-world applications in terms of trustworthiness and ethical use.
Also, I am interested in combining LLMs with other aspects of science, such as LLM + Biology and LLM + Healthcare. I am committed to utilizing AI to solve complex challenges and drive innovation at the intersection of technology and science.
I am willing to explore new possibilities in innovative uses of LLMs. Please don't hesitate to contact me for potential collaborations and opportunities! ☺️
Education
Purdue University
Ph.D. Elmore Family School of Electrical and Computer Engineering
- 2023/8 -- 2028/5 (Expected)
- Adviser: Prof. Jing Gao (opens new window)
Wuhan University
B.Eng. School of Computer Science
- 2019/9 -- 2023/5
- GPA 3.95/4.0, Rank 1/30
Select Publications
Note: {} encloses co-first authors. For full publication list, see here.
SaySelf: Teaching LLMs to Express Confidence with Self-Reflective Rationales
Tianyang Xu, Shujin Wu, Shizhe Diao, Xiaoze Liu, Xingyao Wang, Yangyi Chen, Jing Gao
Large language models (LLMs) often generate inaccurate or fabricated information and generally fail to indicate their confidence, which limits their broader applications. In this work, we present the advanced SaySelf, a training framework that teaches LLMs to express more accurate fine-grained confidence estimates. In addition, beyond the confidence scores, SaySelf initiates the process of directing LLMs to produce self-reflective rationales that clearly identify gaps in their parametric knowledge and explain their uncertainty.
Mixture-of-Domain-Adapters: Decoupling and Injecting Domain Knowledge to Pre-trained Language Models Memories
{Shizhe Diao, Tianyang Xu}, Ruijia Xu, Jiawei Wang, Tong Zhang
Although continued pre-training on a large domain-specific corpus is effective, it is costly to tune all the parameters on the domain. In this paper, we investigate whether we can adapt PLMs both effectively and efficiently by only tuning a few parameters. Our proposed Mixture-of-Domain-Adapters (MixDA) employs a two-stage adapter-tuning strategy that leverages both unlabeled data and labeled data to help the domain adaptation: i) domain-specific adapter on unlabeled data; followed by ii) the task-specific adapter on labeled data. MixDA can be seamlessly plugged into the pretraining-finetuning paradigm.
SHIELD: Evaluation and Defense Strategies for Copyright Compliance in LLM Text Generation
Xiaoze Liu, Ting Sun, Tianyang Xu, Feijie Wu, Cunxiang Wang, Xiaoqian Wang, Jing Gao
Large Language Models (LLMs) have transformed machine learning but raised significant legal concerns due to their potential to produce text that infringes on copyrights, resulting in several high-profile lawsuits. To tackle these challenges, we introduce a curated dataset to evaluate methods, test attack strategies, and propose lightweight, real-time defense to prevent the generation of copyrighted text, ensuring the safe and lawful use of LLMs.
Academic Services
Reviewer
Served as a Conference External Reviewer for PAKDD 2023.
Teaching Assistant
Served as a teaching assistant for various Purdue courses, including ECE 56200 (Data Management) and ECE 36800 (Data Structures).